A new model for AI governance
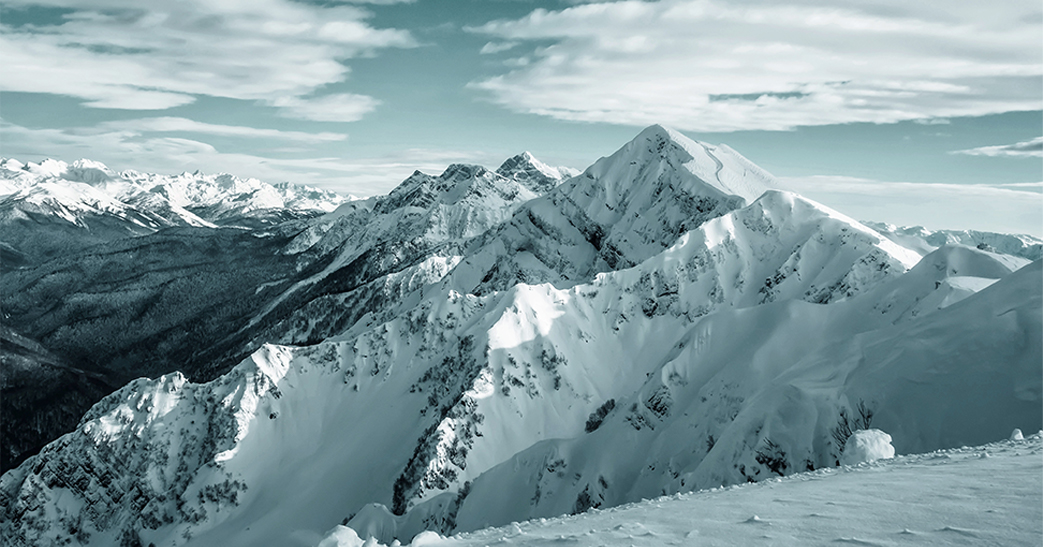
Over the last centuries, humanity has tested various governance models for society and individuals. Checks and balances, separation of powers and democracy to be governed as fair as possible. It’s about not giving too much power to a single entity by having proper governance methods in place.
Over the last centuries, humanity has tested and implemented various governance models for society and individuals. Checks and balances, separation of powers and democracy are ways of making our societies to be governed as fair as possible. It’s about not to give too much power to one single entity or individual by having proper governance methods in place.
Artificial intelligence is set to impact the governance models of both human individuals and societies. In some countries and companies, AI is already partially integrated into political activities and economic decisions. Of course, when a semi-autonomous decision-making process is set in place, explainability and bias become core issues. Recent news about discriminating AI models, such as the two models that Amazon was recently forced to roll back (1,2) or models that can be easily manipulated to produce different results (Google photos mistake humans for gorillas, a colorful patch can make you invisible to AI) prove that it is a theme that is far from being resolved and it can even be a bottleneck on the general adoption and acceptance of AI models.
Precisely because of these concerns, the main-stream AI adoption will most likely take place in the private economy at first. Companies searching for increase in efficiency and applications of customised care at scale already adopt autonomous decision-making models. A fundamental question comes up - “how do I know that AI treats me in a fair way? E.g. certain people are not getting disadvantaged because a model treats them unfairly? A key question in confidential computing.
This question has two main perspectives:
1. The developer side
2. The user side
On the developer side, much research is dedicated to finding ways to establish unbiased models and making them more transparent on their decision logic. This way, practitioners try to get more control over what the model is learning and why it took the decision it took. Of course, this on its own, guarantees neither equal representation nor fairness. Sure enough, bias and manipulation are not model exclusivity; humans are victims of those too. However, the main difference between humans and models is the scale. While a biased opinion of a single human may affect a small subset of any given population. The bias of a model will affect the entire population and always in the same way. A practical example would be a hiring model. While in a human-led hiring process, the presence of e.g. a sexist screener would only affect the resumes that they screen, in a model-led screening process a model with a “sexist bias” would discriminate against the whole pool of resumes and always against the same gender.
Frequent auditing of these models can mitigate this problem. But how can we make sure that the model that “serves” us is the most recently audited one since the only thing we can see is the prediction?
Trusting the black box
In this article, we present an answer to this fundamental question by using applied cryptography. By combining cryptography and machine learning, we can leverage the zero-knowledge proof protocol to verify a model securely and privately. Our method can verify that a prediction has been made from a specific model without revealing any information about the model’s inner workings. The verification is done automatically, and it is based on mathematical trust. With every prediction, the system automatically generates a small proof (in the size of some kilobytes) with which the user/client can easily verify that the result he got came from a specific model ID. If this caught your interest, please reach out to us for a demo presentation.

What does this mean for the industry?
The results of the availability of such a system have a double sided effect. On the one hand, the companies that are serving machine and deep learning models will be able to provide assurances to their users that any autonomous decision-making model has been adequately audited and unbiased. For example, along with the decision to get a particular premium on your insurance you will get a digital fingerprint that proves that the model which provided this decision was a model that has been fairly audited and unbiased to not discriminate against you.
On the other hand, users will become much more empowered as they will no longer need to trust the company or the organisation that serves the model, but rather the mathematics behind the proofs. Hence, by alleviating the need for trust, the users will be able to distinguish between the companies that go the extra length to provide fair decisions and those who don’t, essentially turning the fair and unbiased decision making from a good-to-have to a competitive advantage for the businesses of tomorrow.
References
Related content
Subscribe to Decentriq
Stay connected with Decentriq. Receive email notifications about industry news and product updates.