Differential Privacy as a way to protect first-party data
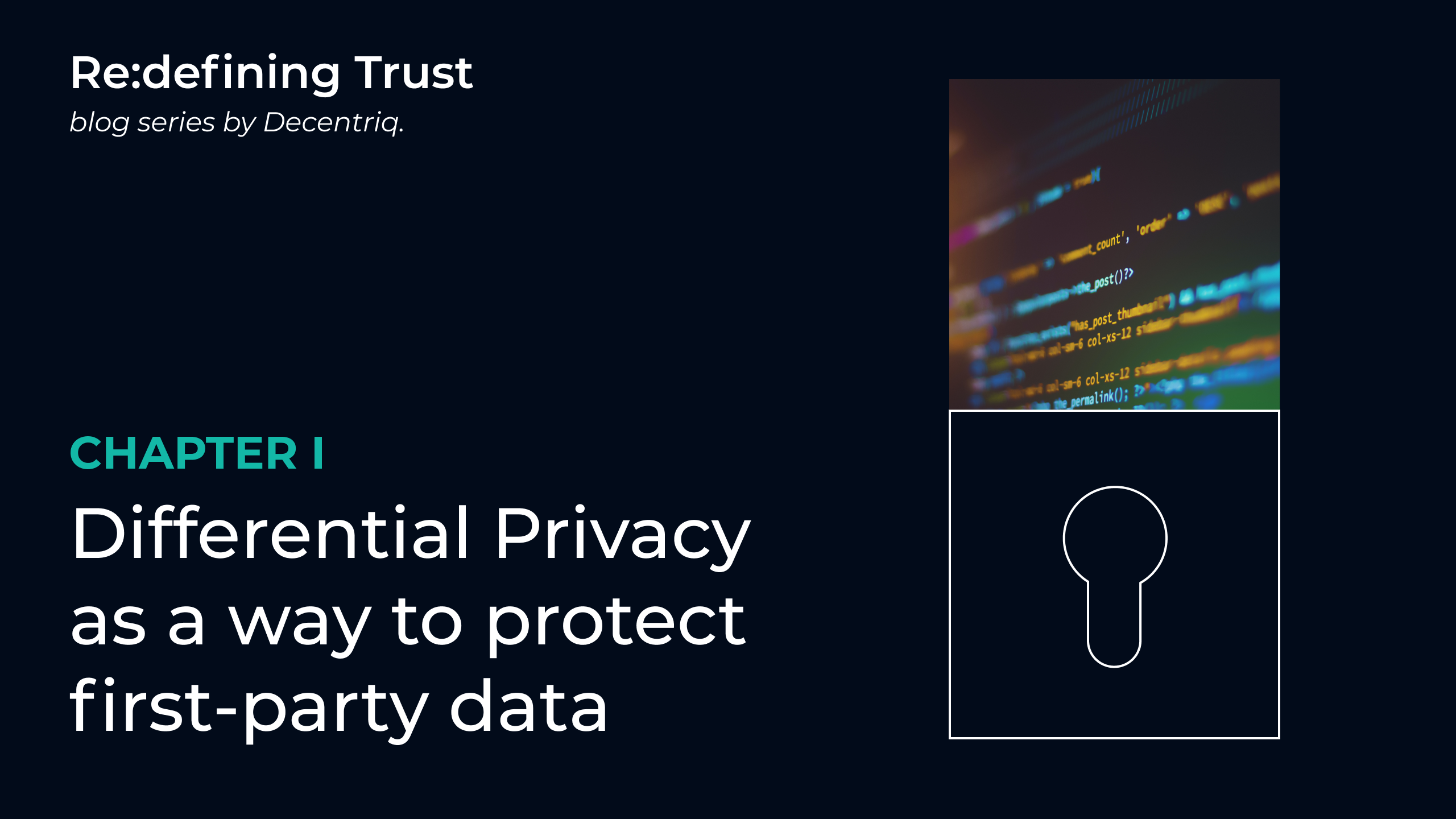
Pseudonymised data is not enough to safeguard privacy or regulations such as GDPR. Differential Privacy approaches privacy in a more quantified way, capturing the essence of the problem.
Privacy concerns from regulators as well from the general public are real. GDPR, third-party cookie deprecation, and the general push towards less intrusive tracking are already affecting the media and advertising landscape. Pseudonymized data is not enough to safeguard privacy or regulations such as GDPR. With third-party cookies going away, matching and augmenting data will become much harder, and keeping first-party data private is already coming into the forefront. In light of this landscape a new technique emerged called Differential Privacy. Differential Privacy approaches privacy in a more quantified way, capturing the essence of the problem at hand: the most accurate ways to use data can leak private information, and the most private data is no data. Differential privacy creates essentially a tuner that allows users to control exactly where they want this tradeoff to lie.
But why is this even needed?
There is one question that any privacy technique is asking itself: Can I learn something about a population without being able to learn anything about an individual? A question which is surprisingly difficult to answer, but also highly important in light of this new privacy-aware social and regulatory landscape.
Take an example, a clothing brand wants to collaborate with a digital newspaper and understand their ad attribution. To do that the need to perform some analysis on the digital newspaper user data in combination with some data of their own. Of course, the platform where they perform that analysis (usually a data clean room) is not supposed to have access to that data. You can make sure that this happens by using a data clean room that has a technology like confidential computing. But this is not the only concern. The newspaper also wants to make sure that the brand is not taking out any individual user information while they are performing their initial analysis. This is where Differential Privacy is coming into play.
In our example above, the newspaper or the brand do not want to reveal any identifying information of their customers to each other. Differential privacy allows them to control the probability of that happening. Notice that we are talking about probability of that happening and not absolutes; that is because in the data privacy domain exists a unique tradeoff; the tradeoff between data utility and privacy. Differential privacy quantifies this tradeoff and gives you tools to control it.
Getting an intuitive understanding of Differential Privacy
To better understand how differential privacy works, we will use the example of the collaboration between the clothing brand and the digital newspaper. The first thing the brand wants to do with the digital newspaper data is understand how many users exist with similar interests as the cloth brand customers. Running these computations without any privacy control could easily allow the brand to single out specific newspaper customers as well as learning more than what they supposed to know about the reading habits of individual brand customers.
What Differential privacy says, is that for a given output, you are limited in how sure you are that a given input could have caused it. This privacy leakage limitation is the result of some noise being added at the process of asking each question. Practically this means that the (noisy) answer of the question brand is asking will be (almost) the same even if any single user was removed from the dataset completely. Consequently the clothing brand can never know if the result they got was coming from a dataset that included a specific user, effectively protecting the privacy of any specific individual. The tuning part comes into play when we talk about the amount of noise you can add to each answer.
The amount of noise is determined by the parameter ε (epsilon). The lower the ε the noisier the data is (and more private). However, a differential private system is not only adding noise, but is able to use the knowledge of ε to optimize the utility of the data by factoring the noise in the aggregate calculations. Determining the right ε in a Differentially private system is a non-trivial task and most of the time because it implies that the data owner is knowledgeable about the privacy risks that the specific ε number entails and what level of risk they are comfortable undertaking . That being said, our client experience has given us some useful defaults that satisfy most use cases.
What makes Differential Privacy different and why should you care?
Privacy that doesn’t ruin your workflow
If we exclude techniques that result in pseudonymization and have proven many times that can be easily broken, providing real privacy guarantees is currently ruining the average data analysis workflow with a lot of aggregation at a “rule of thumb” level and a lot of custom coding to make it happen. And even then, companies cannot be sure that they chose the best possible tradeoff between privacy and utility, resulting either in private information leaking or unusable data. Differential privacy, exactly because it quantifies this tradeoff, is able to be incorporated into existing workflows making existing queries and ML models private on the background without requiring extensive re-writes.
Future proof - today
As we touched upon before, regulation is becoming more and more prominent in ad-tech. And while the laws themselves rarely mention technologies, GDPR is operating under the “best in class” or “state of the art” model where organizations have to put effort into using the latest technological advancements on privacy and security. Differential privacy is the de-facto state of the art for data privacy as it is the most rigorously mathematically proven method for maintaining privacy. This “privacy tuner” that differential privacy is providing is thorough enough to cover any potential regulation needed now as well in the future.
Differential Privacy is not a silver bullet
Privacy in general is not a domain that has silver bullets. Differential Privacy allows organizations to take more informed decisions about their data privacy, but the privacy/utility trade off still exists. So, the most important limitation of differential privacy is that it does reduce the accuracy of an answer. The same random noise that protects data about an individual also makes the final answer noisier. This makes it more appropriate for questions that are fundamentally of a statistical nature where you can accept some natural variation like market research or clinical trials, as opposed to areas where an exact total is necessary like accounting or billing.
The more possible answers to the question there are, the more noise there will be. This means you will need more data for the signal to rise above the noise. So asking a very specific question like “Did this ad campaign increase sales?” or “Does this drug prevent disease?” can get good answers with pretty small sample sizes, not much larger than you would need to get a convincing answer anyway. A more general question with hundreds of possible answers like “what order did people see my ads in” will have a lot of noise because there will be some noise for every possible answer. In any case though, there will be more noise than if you didn’t use differential privacy, so you will need to collect more data to get a statistically significant answer or accept slightly less accurate results.
Another important limitation of differential privacy is that in order to get very strong guarantees you need to use a budget that limits how many questions can be asked of the same data set. This is usually not a large limitation for systems that ask well defined questions or run every day – they can be designed to ask the right number of questions with the right amount of noise for each question. It matters much more for “exploratory” or other custom analysis, where you may not know the total number of questions in advance, so it is hard to predict how much noise each answer needs to have. This is especially challenging for open access or other situations where there is little reputation risk for someone trying to mount an attack – the system needs to keep a tight budget to protect against attackers but that can come into conflict with the budget necessary for valuable legitimate uses.
A differentiator for brands and publishers alike
While usually privacy preservation is not value-generating on its own, having in place a system that guarantees both security and privacy at all times can become a powerful accelerator. We’ve seen multiple exciting use cases based on collaboration of first-party data to fall into inertia due to uncertainty over data security and data privacy. Decentriq’s data clean rooms offer a hands-off approach to both these areas, allowing combine first party data that was impossible before, and draw actionable insights without you or your data partners ever worrying about data security or privacy. Differential privacy is the perfect tool for creating behind-the-scenes data infrastructure automating a process that would otherwise deem a project non-viable.
References
Related content
Subscribe to Decentriq
Stay connected with Decentriq. Receive email notifications about industry news and product updates.