The key to secure LLMs is hidden in confidential computing

Request a live demo
Want to see what else data clean rooms can do? Have a specific use case in mind? Let us show you.
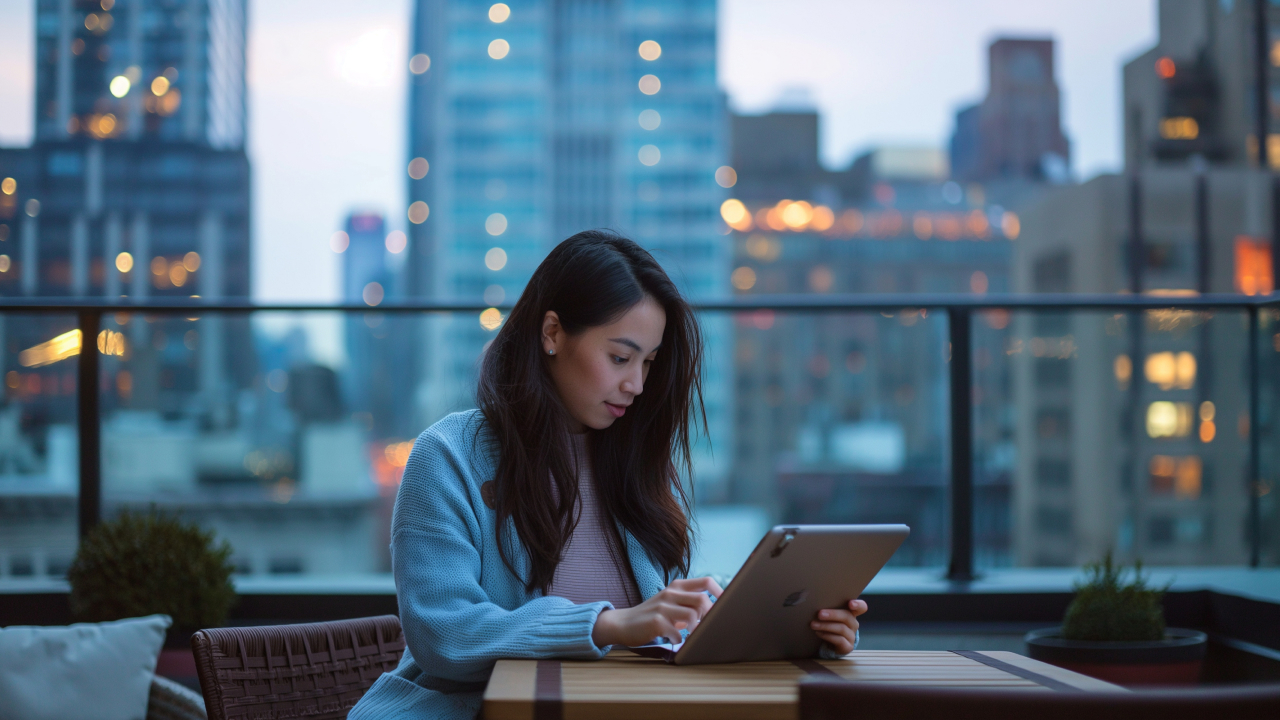
Back in 2022, we wrote a blog about the Secure Enclave Processor that Apple first introduced in the iPhone 5S. This technology was seen as the initial step towards creating a more privacy-preserving computational infrastructure.
Fast forward to this week: 10 years after the release of iPhone 5s, Apple launched Private Cloud Compute, aimed at enhancing the security and privacy of large language models (LLMs) and their Apple Intelligence, effectively closing the circle of secure computing from device to the cloud. While Apple is the first to publicly commit to this security model, they are not alone: OpenAI is also speaking publicly about confidential computing, as detailed in their blog about AI secure infrastructure.
The umbrella term for this suite of technologies is confidential computing, a technology which we’ve been working on for some time at Decentriq. Our data clean rooms are the only data analysis platform deeply integrated with confidential computing, allowing our users to combine and analyze even their most sensitive data in a protected environment. The benefit is simple: Neither Decentriq nor the cloud provider have access to user data at any point, even if it’s processed in our infrastructure. It combines the flexibility and scalability of the cloud with the security of an on-premise installation.
But Apple only showed how this technology can be used to protect your personal data as you interact with your Apple device. What about the rest of your sensitive data?
Your data at the websites, hospitals, and doctors you visit is going to eventually be processed by an LLM as well. We see that demand rising steadily. Over the past six months, every time we speak about data clean rooms and confidential computing, one of the first questions is “Can I run LLMs inside it?”. The answer is “Yes”.
For the first time, we present an open-source LLM running in a confidential computing environment in our data clean rooms.
But why has confidential computing now become a such an important point of discussion at Apple and OpenAI? We argue it is because the next step in AI is personal assistants with deep knowledge of your (sensitive) data. These assistants are a much bigger honeypot for all types of adversaries.
Before the advent of LLMs, data breaches typically exposed static datasets—emails, documents, financial records. While concerning, the scope of these leaks was often limited to the data itself. So if you were not processing sensitive data, existing approaches where sufficient. However, with assistants like the new Siri or other LLM agents, the sensitive user data comes pre-packaged with a model that is able to interpret and combine them. No one wants a model which knows their health history to fall into the wrong hands.
And as is usual in security, bigger honeypots come with more actors tempted to tap into them. For many organizations and people, these actors include the LLM providers themselves as well as the cloud infrastructure that is running them. Users find it hard to take providers at their word that they won’t use this data, especially with multiple ongoing legal cases around IP violations for training. This concern is naturally more prevalent in cases where the user shares very sensitive information such as healthcare data. With confidential computing (or Private Cloud Compute), the users do not trust the cloud or the company that they won’t use their data for training or even reveal it when subpoenaed. They simply can’t.
To showcase the future of handling sensitive data, we present a preview of our platform’s new feature that leverages open-source LLMs. This feature allows users to combine their sensitive data and query it, all within the secure confines of data clean rooms and under the encryption guarantees provided by confidential computing.
Disclamer: Yes, had to tune the system prompt quite a bit to generate those responses 🙂
We are already seeing a lot of exciting use cases coming from our users. From faster and easier patient data harmonization across medical sites in healthcare, to even better AI-assisted data exploration and lookalike audience creation in media and advertising.
As Apple's Private Cloud Compute marks a significant leap in securing personal data interactions, here at Decentriq, our data clean rooms take this innovation a step further by safeguarding sensitive external data. This enables organizations to confidently deploy AI, including LLMs, across a spectrum of use cases while maintaining the highest standards of data privacy and security.
References
Request a live demo
Want to see what else data clean rooms can do? Have a specific use case in mind? Let us show you.
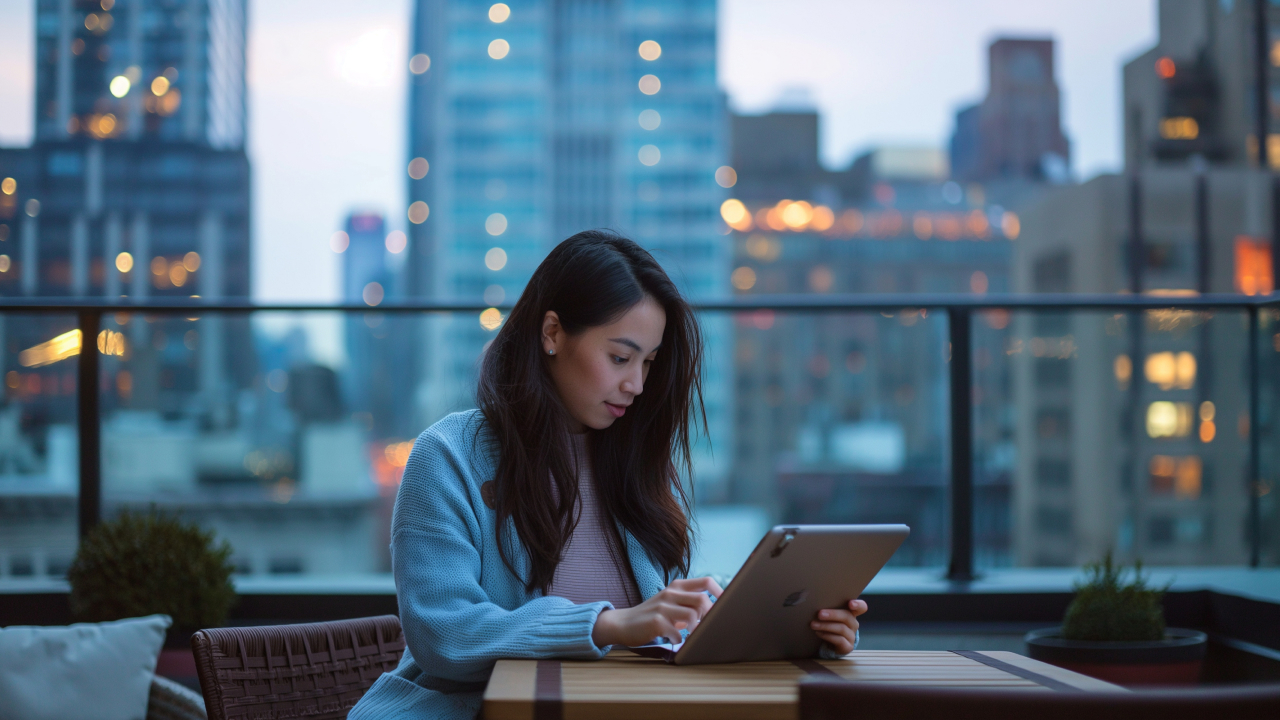
Related content
Subscribe to Decentriq
Stay connected with Decentriq. Receive email notifications about industry news and product updates.