6 challenges in advancing real-world data use in healthcare — and their solutions
.jpg)
Whitepaper: Unlock the value of real-world healthcare data with confidential data clean rooms
As the amount of healthcare data from real-world settings grows, how can care providers and life sciences companies use this data to advance research and treatment while protecting sensitive patient information?
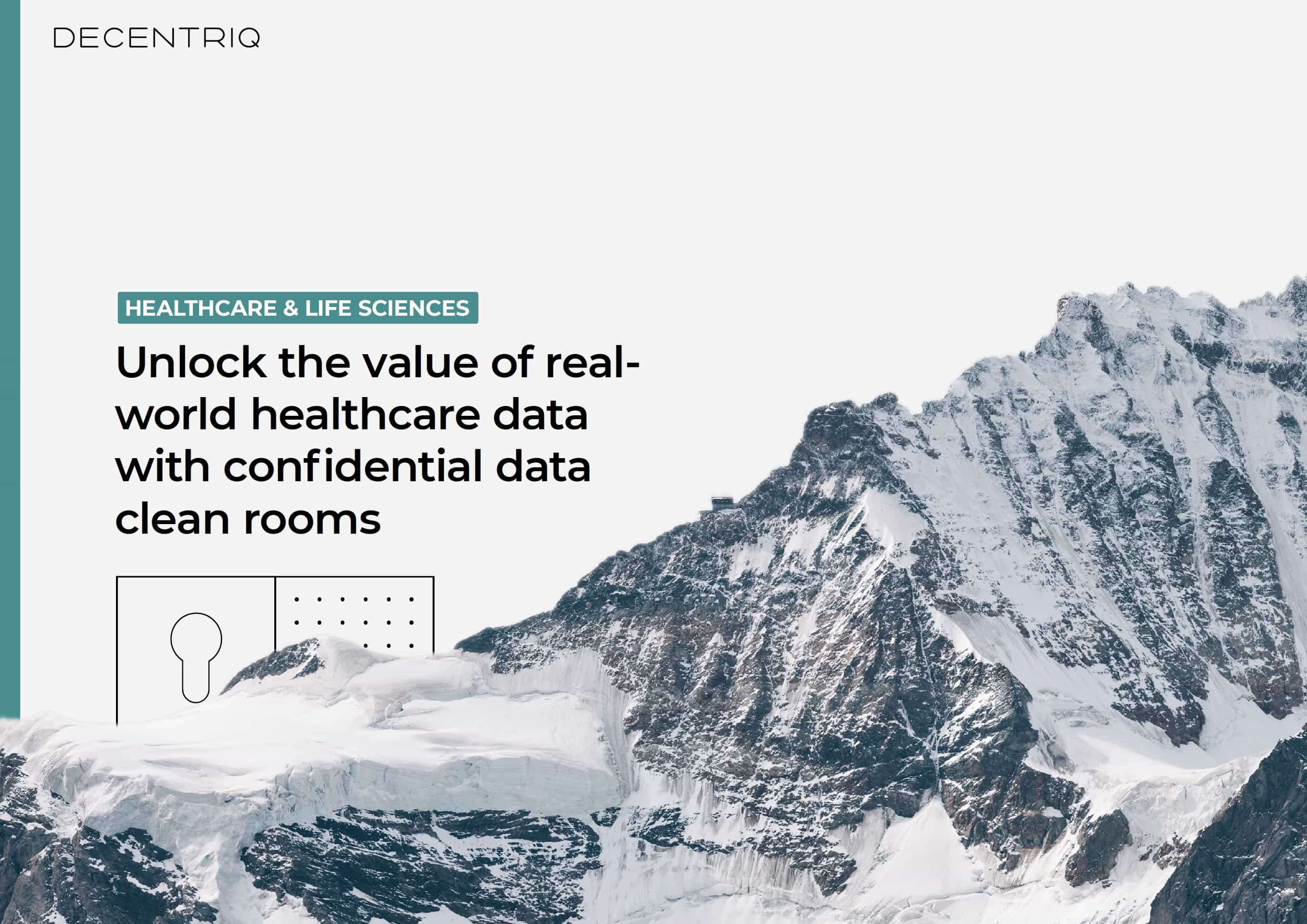
The sheer volume of healthcare data is staggering, with healthcare provider data expected to grow to 8,809 exabytes by 2026.
This data is also becoming increasingly necessary. In fact, by the end of 2020, 90% of new US Food and Drug Administration drug approval submissions included real-world data (RWD). The European Medical Agency expects to see real-world evidence (RWE) in regulatory submissions and post-safety evaluations by 2025.
Clearly, the ability to access and collaborate on RWD has huge potential for all healthcare stakeholders. However, as demand for RWD increases, the existing system of siloed, fragmented data and slow-moving partnerships will be unable to keep pace.
This article outlines the most common challenges standing in the way of collaborations involving RWD and how the right technologies can solve them.
%20(1).png)
Challenge 1: Heterogeneous data formats
Big data has the potential to support unprecedented opportunities and use cases within healthcare. RWD partnerships are already helping organizations reduce the cost of care, increase access to treatments, and improve outcomes. Expanding these partnerships into large-scale, ongoing collaborations that create an ecosystem of connected data could transform healthcare.
Imagine combining information from Internet of Things (IoT) devices with medical records to understand how lifestyle factors impact risk and disease progression. Or pooling siloed data together to identify seemingly unrelated symptoms that could help diagnose rare diseases faster. Data collaboration solutions accommodating heterogeneous data types and formats can lead to more alignment between drug development, patient need, and increased coverage from payors.
Challenge 2: Varied rules and regulations
The governance of personally identifiable data varies from country to country. Even under the EU General Data Protection Regulation (GDPR), there are differences in implementation and interpretation across member states.
These differences lead to uncertainty and risks in navigating the legal landscape around collaborating on health data. This can deter manufacturers of digital health products and providers of digital health services from expanding into new markets and limit cross-border collaborations.
Opting for a solution with an end-to-end encrypted approach for data collaborations enables full guarantees for privacy and legal compliance.
Challenge 3: Fear around how data and insights will be used
Beyond rules and regulations that protect patient privacy, organizations also need to consider how insights will be used. Medicare Advantage, a U.S.-based private health plan, is allegedly using artificial intelligence algorithms to reduce or deny coverage, according to a STAT investigation. In one example, the insurer cut off coverage of hospital care when a woman exceeded the predicted recovery time, despite medical records showing she was unable to return home.
To participate in data collaboration, organizations need to understand how sensitive data will be used to avoid even the perception that sensitive information was leaked or misused. This is critical in protecting the organization’s reputation, as well as building and maintaining patient trust.
Data custodians should retain control of their data at all times and have transparency around access rights, planned analysis, approved outputs, and how those outputs will be used.
To provide this level of transparency and control, partners should choose collaboration methods where raw data is never shared with or accessible to external parties.
Challenge 4: Protecting intellectual property
Privacy concerns also apply to proprietary information that might be exposed. Companies rightly want to protect their information, especially if they are training algorithms for future products and services. This goes beyond legal requirements around patient privacy and raises the stakes in choosing collaboration methods that fiercely protect privacy for all parties.
Challenge 5: Expensive, time-consuming collaborations don’t scale
Historically, establishing data collaborations has been hard. This involves finding partners who are able and willing to partner on their sensitive data, setting up complicated frameworks, and working through data protection requirements.
As a result, collaborations can take at least a year to establish, which is wildly at odds with the pace of technology and the demand for better treatments faster.
Collaboration agreements and technology solutions are so unique to the specific partners that they become single-use solutions. Every new partnership requires another round of time-consuming negotiations and costly setup. This discourages collaboration with more diverse partners that could provide broader data sets.
As brought up in challenge 2, this process can be shortened significantly by selecting a zero-trust solution. Using confidential computing and other advanced privacy technologies can provide hard proof that data remains confidential, facilitating compliance with data protection regulations. This eliminates the need for repeated compliance agreements and documentation.
Using solutions employing these measures can reduce the time needed to establish collaboration frameworks by 90%.
Challenge 6: Rigid solutions impact data usability
The above challenges can result in very rigid collaboration methods and limited data sets. For example, some patient data might be excluded due to privacy concerns, or hospitals might opt out of the study if the barriers seem too high. Reducing the available data or severely limiting how it can be used restricts the accuracy and scope of the RWE that can be derived.
Collaborators need flexible solutions that allow participants to manage the tradeoff between privacy and utility based on the scope of the study and the comfort levels of the data custodians.
These solutions should be easy to use and able to guarantee that outputs won’t reveal private or confidential information, even if some participating organizations only contribute a few cases. Enabling organizations of all sizes to contribute results in more complete data sets.
Decentriq’s data clean rooms enable healthcare and life sciences organizations to overcome these challenges and concentrate on the important work of using data to make healthcare more equitable, affordable, and personalized.
Learn more about how to take advantage of the many opportunities offered by collaboration on RWD in our whitepaper “Unlock the value of real-world healthcare data with confidential data clean rooms”.
References
Whitepaper: Unlock the value of real-world healthcare data with confidential data clean rooms
As the amount of healthcare data from real-world settings grows, how can care providers and life sciences companies use this data to advance research and treatment while protecting sensitive patient information?
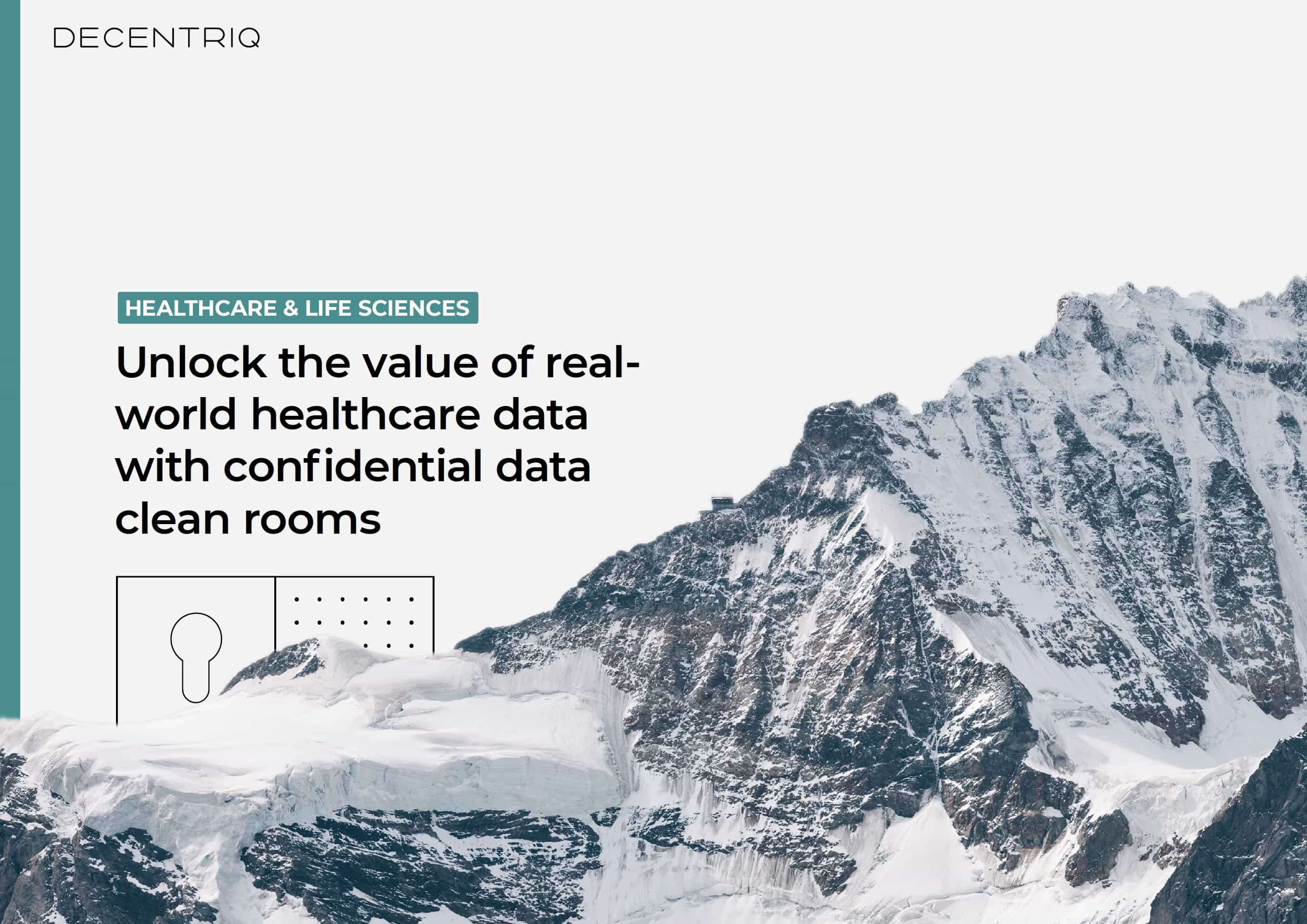
Related content
Subscribe to Decentriq
Stay connected with Decentriq. Receive email notifications about industry news and product updates.