What are privacy-enhancing technologies (PETs)?
.jpg)
Find out about the most important types of privacy-enhancing technologies and how they help maintain the confidentiality and integrity of data.
Your guide to reducing wasted ad spend using first-party data
An estimated 23-56% of ad spend is currently wasted (and that’s before third-party cookies are completely deprecated). So how can brands ensure they’re reaching their ideal audiences at a time when consumers expect more personalized — yet privacy-preserving — advertising experiences than ever before?
.jpg)
Privacy-enhancing technologies (PETs) are technologies, tools, techniques, and practices designed to protect individuals' privacy. They achieve this by safeguarding personal data during storage, processing, and transmission. And with 94% of consumers expecting companies to protect their data and data breaches costing businesses an average of$4.45 million per incident, organizations must find ways to harness data without compromising privacy. As regulations tighten, PETs are becoming acritical solution for ensuring compliance and maintaining trust.
Sometimes also referred to as privacy-preserving technologies or data protection technologies, PETs include methods like encryption, anonymization, access controls, and solutions such as differential privacy, synthetic data generation, and confidential computing. They help organizations and individuals maintain control over their data and mitigate privacy risks in an increasingly data-centric world.
Here, we discuss what privacy-enhancing technologies are, how they work, and why they're essential tools in today’s digital toolkit.
Privacy-enhancing technologies: Definition
Privacy technologies encompass a wide group, but each type of privacy-enhancing technology has a similar aim: To act as a safeguard, ensuring that personal information remains private, even when being used for data collaboration and analysis.
The Royal Society has a brief explainer video that sheds some light on the topic:
Types of privacy-enhancing technologies
Synthetic data
Synthetic data allows organizations to generate artificial data that closely mimics real-world data, while still preserving privacy.
Organizations can safeguard sensitive information by generating synthetic datasets. These synthetic datasets closely resemble real data, encompassing not only the same shape but also similar statistical qualities. While they lack private details, they maintain correlations and patterns found in real data. This enables companies to conduct analyses and develop machine learning models without the risk of data exposure.
For example, in a well-designed synthetic dataset, it might be possible to observe a correlation between age and heart disease, preserving the statistical characteristics crucial for accurate analysis.
Use cases:
- AI and machine learning – Synthetic data is frequently used in AI and machine learning to create training datasets when real data is limited or too sensitive to use.
- Software testing and development – Developers use synthetic data to test applications or systems without risking exposing real customer data, ensuring that privacy is maintained.
Example:
A healthcare company uses synthetic patient data to develop a new predictive algorithm for diagnosing diseases. The synthetic dataset reflects the characteristics of real medical records but doesn't expose any actual patient information, protecting privacy while still allowing the algorithm to be tested and refined.
Differential privacy
Differential privacy is a mathematical method used in data analysis. It works by introducing randomness or noise into query responses, making it harder to pinpoint individual data points.
However, not all noise-adding techniques qualify as differential privacy. Differential privacy is specifically about determining the precise amount of noise needed to achieve statistical privacy assurances.
Differential privacy employs aggregation to balance data analysis with privacy preservation. This technique involves summarizing and generalizing data to derive meaningful insights while protecting individual privacy.
Practical use cases:
- Census data – Governments can apply differential privacy to their census data, ensuring that individuals cannot be identified through detailed demographic information, even when data is broken down into small geographic units.
- Consumer behavior analysis – Companies can analyze customer behavior patterns across different regions or demographic groups without revealing any individual’s data.
Learn more about differential privacy for media and advertising.
Example:
The U.S. Census Bureau applied differential privacy to protect the privacy of respondents. When the census data is made publicly available, the noise ensures that no one can use the data to identify specific households or individuals.
Confidential computing
Confidential computing enables data processing within secure enclaves. This innovative approach prevents unauthorized access to data during computation, offering a new level of security in data processing and analysis.
Confidential computing keeps sensitive data safe even during use with two key data security methods: isolation and remote attestation. The former safeguards sensitive information while in use, while the latter verifies this protection and what the data will be used for before computation even begins.
Use cases:
- Cloud computing – Confidential computing enables organizations to securely process sensitive data in the cloud without risking exposure to the cloud provider or external threats.
- Healthcare and financial services – These industries use confidential computing to perform computations on highly sensitive data (e.g., medical records or financial transactions) in environments where privacy and security are paramount. These industries use confidential computing to perform computations on highly sensitive data (e.g., medical records or financial transactions) in environments where privacy and security are paramount.
Example:
Swiss banks used confidential computing from Decentriq to collaborate and gain insights into cyberthreats. The result was they were able to detect new phishing campaigns, identify common patterns and compare the phishing defense of all participating organizations.
Homomorphic encryption
Homomorphic encryption enables computations on encrypted data without decrypting it first. This ensures data privacy while still allowing meaningful operations to be carried out on the encrypted information.
Use cases:
- Secure data analytics – Companies can perform data analysis on encrypted datasets without revealing the underlying sensitive information.
- Financial calculations – Homomorphic encryption is used in the financial industry to perform calculations on encrypted transactions, ensuring that sensitive financial information remains protected.
Example:
In digital advertising, homomorphic encryption enables advertisers to analyze encrypted user data without accessing personal information. This allows for the delivery of personalized ads while maintaining user privacy. For example, an advertising platform can process encrypted user preferences to match relevant ads, ensuring that sensitive data remains confidential throughout the process.
Secure multiparty computation
Secure multiparty computation (SMC) relies on cryptographic protocols using encryption and mathematical techniques to enable multiple parties to jointly compute a function over their individual inputs while keeping those inputs private. It ensures that no party learns anything beyond the output of the computation, even in the case of participants who follow the protocol correctly but might attempt to learn additional information from the received data.
Use cases:
- Collaborative research – Multiple institutions can securely analyze combined datasets without revealing their individual data to each other.
- Joint business intelligence – Businesses can collaborate on shared market analyses, pooling their data for better insights, while maintaining the confidentiality of their proprietary datasets.
Example:
A group of healthcare providers wants to collaborate on a new research project using patient data, but each institution must protect its own data due to privacy concerns. Using SMC, they can securely compute a joint analysis of treatment outcomes while ensuring that no party can access the sensitive data of the others.
Federated learning
Federated learning is a decentralized machine learning approach. Here, a model is trained across multiple decentralized devices or servers holding local data samples, without exchanging them. Instead of sending raw data to a central server, only model updates (gradients) are communicated, preserving data privacy.
Use cases:
- Mobile AI – Federated learning enables mobile devices to improve features like predictive text, image recognition, or voice assistants without sharing sensitive user data with a central server.
- Healthcare AI – Hospitals and healthcare institutions can collaborate on training AI models for medical diagnosis without exchanging patient data, preserving privacy while developing more robust models.
Example:
Google uses federated learning in its Gboard keyboard to improve predictive text functionality. The data remains on individual users' devices, and only updates to the machine learning model (based on their local usage) are sent to Google’s servers, ensuring privacy.
Trusted execution environments
Trusted execution environments (TEEs) are secure hardware or software environments within a computer system. They provide a secure and isolated area for executing sensitive code or operations. They protect code and data within them from external tampering, even from the operating system or other software layers.
Enclaves and trusted execution environments are a key part of confidential computing and are broadly interchangeable terms. They typically imply that the environment is hardware-based. A few rare exceptions of software-based “enclaves” exist, but they provide less robust security.
Use cases:
- Cloud data processing – TEEs enable secure data processing in the cloud, where users can compute on sensitive data without exposing it to the cloud provider or other parties.
- Secure financial transactions – TEEs are used in the financial sector to securely process transactions and other sensitive operations while keeping the underlying data protected.
Example:
A financial institution may use TEEs in its cloud infrastructure to securely process credit card transactions. Even if the cloud provider or server is compromised, the transaction data remains encrypted and secure within the TEE, preventing unauthorized access.
"Encryption and anonymity, today's leading vehicles for online security, provide individuals with a means to protect their privacy, empowering them to browse, read, develop and share opinions and information without interference [...]." — David Kaye, former UN Special Rapporteur on freedom of expression
What are the benefits of privacy-enhancing technologies?
With the rapid growth of digital technologies, individuals and organizations are sharing and processing an unprecedented amount of personal data. This creates new opportunities, but also significant risks. Privacy-enhancing technologies are essential for addressing these risks, enabling secure data processing, maintaining privacy, and ensuring compliance with ever-evolving privacy regulations. And adoption progress shows no signs of stopping, with the global PET market estimated to grow to USD 28.4 billion by 2034.
Below, we explore the key reasons why PETs are crucial, both for individuals and organizations.
For individuals:
Enhanced privacy
As more personal data is shared online, maintaining privacy has become a significant concern. PETs help individuals control how their personal data is used, ensuring that they retain ownership and protect their identities. Without PETs, individuals are at risk of having their data exposed, leading to identity theft, fraud, or unwanted surveillance.
Use case:
A consumer uses a secure online payment platform that employs tokenization. Their credit card information is tokenized, meaning that the sensitive data is never stored or transmitted in its original form, ensuring privacy during the transaction process.
Example:
When signing up for a new online service, users may choose to use a pseudonymization technique to keep their real name and contact details secure. This means the platform uses this pseudonymized data for its operations, reducing the risk of exposing the user's actual identity if a breach occurs.
Reduced risk of data breaches
Data breaches are one of the most significant threats to personal privacy. When data is exposed, individuals can become victims of financial theft, fraud, or identity theft. PETs like encryption, differential privacy, and secure multiparty computation help minimize the risk of unauthorized access to sensitive data, reducing the impact of potential breaches.
Use case:
An individual’s personal health information is encrypted before being stored in a hospital’s database. Even if the database is hacked, the data remains encrypted, preventing unauthorized access to sensitive health records.
Example:
When using an online banking app, customers' account details and transaction histories are encrypted using homomorphic encryption. This ensures that even if a hacker intercepts the data while it's in transit, it remains unreadable and secure.
Improved trust in digital platforms
The growing concern over privacy issues has made trust a key factor in individuals' decisions to use digital platforms and services. By using PETs, companies can demonstrate their commitment to protecting customer data, fostering greater trust. This is especially important as consumers become more conscious of how their personal information is being used.
Use case:
A company incorporates differential privacy into its analytics platform, allowing it to process user data for insights without exposing any individual’s information. This builds consumer trust, as users know their data is being handled securely and anonymously.
Example:
A tech company that offers online health tracking services uses federated learning to train its AI models. The company guarantees users that their sensitive health data never leaves their device, which encourages users to trust the platform with their personal health information.
Better online experiences
When data privacy is ensured, individuals can enjoy more personalized and engaging online experiences without sacrificing their privacy. By leveraging PETs, businesses can deliver tailored recommendations, services, and products, all while keeping personal information secure.
Use case:
A music streaming platform uses federated learning to improve its recommendation algorithm based on users' listening habits. The platform can offer a personalized experience without sharing users' private data, ensuring that their preferences remain secure.
Example:
A user of a fitness app experiences tailored workout plans, based on their activity data stored on their device. The app uses homomorphic encryption to compute insights locally on the device, ensuring that all data stays private and never leaves the user’s phone.
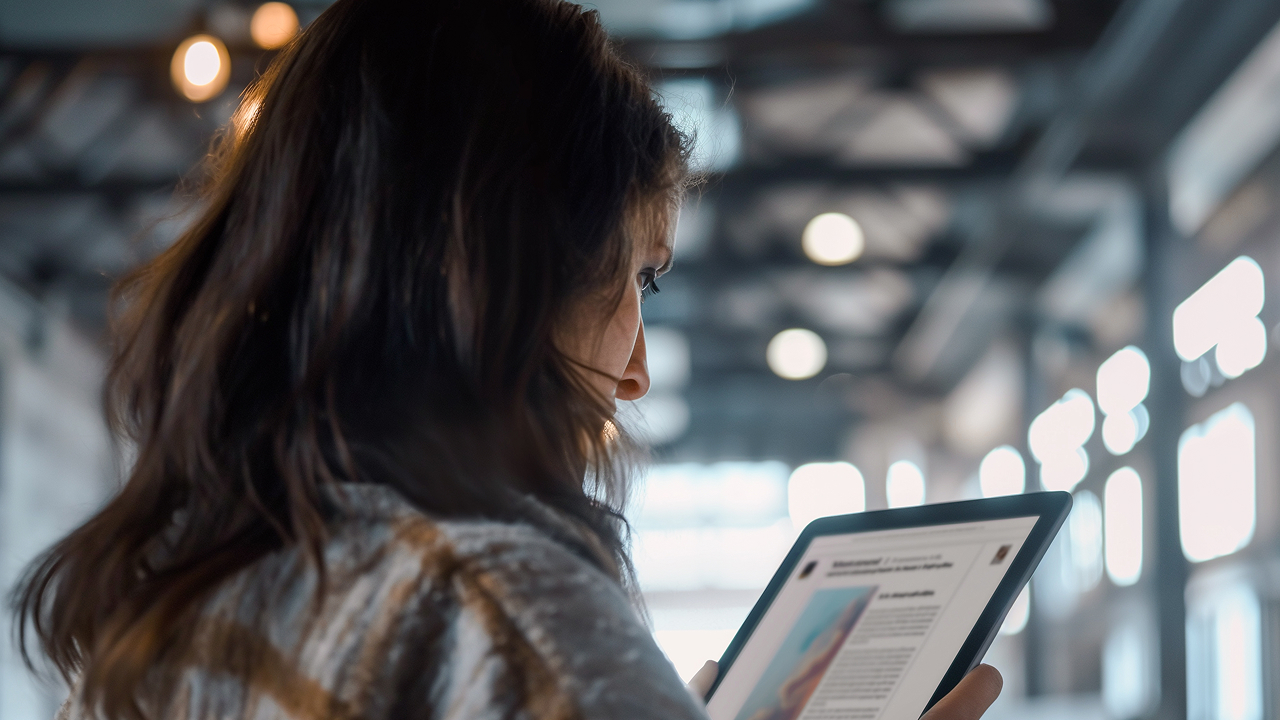
For organizations:
Compliance with privacy regulations
As privacy regulations become stricter globally, organizations are required to implement robust privacy protection measures for personal data. PETs are crucial for ensuring compliance with data protection laws such as the General Data Protection Regulation (GDPR), Health Insurance Portability and Accountability Act (HIPAA), and California Consumer Privacy Act (CCPA). PETs can help organizations can avoid legal consequences, fines, and reputational damage.
Use case:
An e-commerce company processes customer data to improve its services. It uses encryption to protect personal information, ensuring that it complies with GDPR requirements, which mandate the secure storage and processing of customer data.
Example:
A healthcare provider uses data collaboration technology based on privacy-preserving technology to collaborate on the data for research purposes. This ensures compliance with HIPAA, which protects patient confidentiality while allowing valuable data to be used for medical advancements.
Secure data collaboration
Organizations often need to collaboration on data with third parties, such as partners, vendors, or researchers. PETs allow for secure data collaboration without exposing sensitive information. This promotes innovation while preserving privacy. Secure multiparty computation and federated learning, for example, enable multiple parties to collaborate on data analysis without revealing their individual datasets.
Use case:
A pharmaceutical company collaborates with multiple research institutions to develop a new drug. By using secure multiparty computation, they can share and analyze research data without disclosing sensitive patient data or proprietary research findings to the other parties.
Example:
A multinational company uses federated learning to train AI models on data collected from various international branches. Each branch processes the data locally on its devices, contributing only model updates rather than raw data, ensuring that no sensitive customer data crosses borders.
Data minimization
One of the key principles of privacy regulations like the GDPR is data minimization, which dictates that only the minimum amount of data necessary fora specific purpose should be collected and processed. PETs enable organizations to work with data more efficiently and securely, without storing or processing unnecessary personal information.
Use case:
A financial institution uses tokenization to reduce the storage of sensitive information such as full credit card numbers. Tokenization allows the institution to perform transactions without ever storing the actual credit card details, minimizing the risk of exposure.
Example:
An online retailer uses pseudonymization to anonymize user data for targeted marketing campaigns. This allows the company to use the data for personalized offers without revealing personal information like names or addresses.
Data misuse prevention
PETs provide a framework to prevent the misuse of data. By applying encryption, homomorphic encryption, and other privacy-preserving techniques, organizations can ensure that data is only used for the intended purpose and by authorized parties, reducing the risk of data being misused, sold, or shared inappropriately.
Use case:
A social media platform uses trusted execution environments to process users' personal data for targeted advertising. By using TEEs, the platform ensures that advertisers can only access the aggregated, anonymized data and not any personally identifiable information.
Example:
A government agency working with sensitive citizen data employs secure multiparty computation to collaborate with external contractors. The contractors are only given the necessary aggregated results, preventing the misuse or leakage of confidential individual data.
Innovation and data use
While data privacy is critical, organizations also need to use data effectively to innovate and improve services. PETs allow organizations to utilize data for analysis and insights without sacrificing privacy. By adopting PETs, organizations can unlock new opportunities for innovation while adhering to privacy standards.
Use case:
A research organization uses synthetic data to test and develop new AI models. By using synthetic data, they can advance their research without exposing any real personal data, leading to innovation while maintaining privacy.
Example:
A tech company develops a recommendation engine using federated learning. The company can provide personalized suggestions to its users without ever accessing their sensitive data, ensuring privacy while continuing to innovate and improve the service.
Reduced legal and financial consequences
Failure to protect personal data can result in significant financial penalties and damage to an organization's reputation. PETs help mitigate this risk by ensuring that data is handled securely and in compliance with privacy laws, ultimately protecting organizations from costly lawsuits, fines, and reputational harm.
Use case:
An online marketplace implements differential privacy to aggregate data for customer insights while ensuring that no individual’s information is exposed. This helps the company stay in compliance with privacy regulations like GDPR, avoiding penalties.
Example:
A financial services firm adopts homomorphic encryption to secure sensitive client data in their cloud-based systems. This encryption ensures that even if the data is intercepted during processing, it remains unreadable, protecting the company from potential financial penalties.
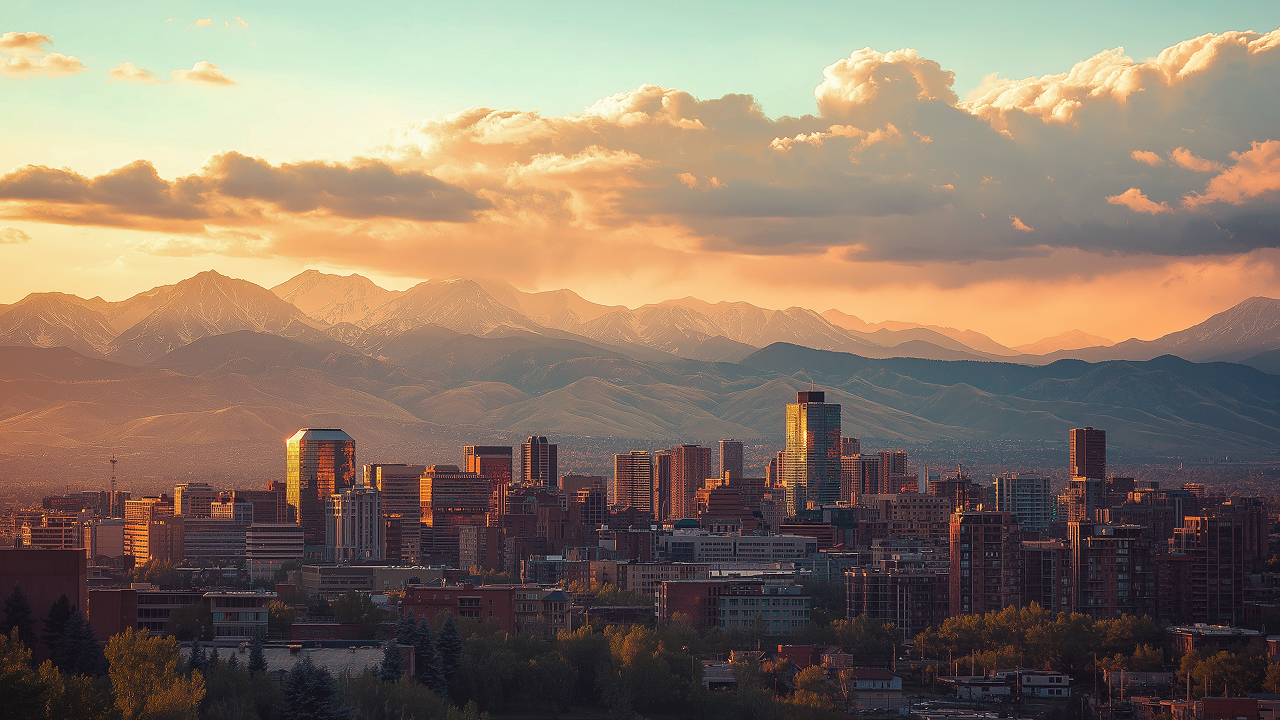
Privacy-enhancing technology examples in action
Use cases for PETs are most commonly found where organizations must safeguard personal data while still enabling valuable data-driven insights. Some examples of verticals that use privacy-enhancing technologies are:
Healthcare
Healthcare providers, researchers, and institutions use PETs to collaborate on and analyze patient data while preserving patient privacy.
For example, theiCARE4CVD consortium needed sensitive, high-quality patient data to train its AI models, but faced challenges around patient privacy and compliance with international health regulations. They also needed to protect the competitive advantages of their proprietary clinical trial data. As a result, iCARE4CVD required a platform running on PETs that could: encrypt data, preserve privacy in generated results, and support machine learning analysis
Read more about privacy-preserving technology and its ability to make real-world healthcare data available for collaboration that results in improved patient outcomes.
Financial services
PETs help protect financial data during transactions, fraud detection, and risk assessment while adhering to regulatory requirements.
For example, Decentriq’s data clean room technology built on PETs played a pivotal role in a Swiss federal pilot project aimed at enhancing the cybersecurity of the nation's financial sector. Built on confidential computing, the Decentriq platform allowed secure and encrypted collaboration between financial institutions like the Swiss National Bank, SIX, and Zurich Cantonal Bank without exposing sensitive data. This enabled participants to identify phishing threats, detect patterns, and compare defenses across organizations while maintaining strict data privacy.
Digital advertising
It's no secret that siloed data and regulatory constraints have the potential to create hurdles to first-party data activation. In this landscape, the challenge lies in enabling seamless, compliant collaboration across platforms.
PETs enable personalized advertising without exposing individuals' personal information, allowing ad targeting without privacy infringement.
Learn how Samsung used privacy-first data matching using tech built on PETs to unlock new opportunities to analyze and collaborate across previously disconnected platforms, reach millions of customers across publishers and drive personalized engagement. You may also be interested in reading about how exactly these technologies enable enhanced ad targeting effectiveness while preserving privacy.
Market research
Companies can collaborate with anonymized data, preserving individual privacy while gaining insights into market trend sand consumer behavior.
For example: IM Associates, a leading healthcare data insights provider, faced challenges with conducting benchmarking studies due to its reliance on third parties for data anonymization. This traditional approach not only introduced friction and raised concerns about data security and participant turnover but also hindered the benchmarking process.
Discover how using Decentriq’s technology built on PETs as a foundation enabled IM Associates to conduct confidential benchmarking studies with unprecedented security and efficiency, liberating them from the constraints of traditional anonymization methods.
Cybersecurity
PETs help protect sensitive security data, detect threats, and analyze network traffic without exposing vulnerabilities. In practice, organizations are increasingly turning to PETs to collaborate on threat intelligence and behavioral analytics without sharing raw data—especially when that data includes confidential logs or user information.
For example, secure multi-party computation can enable multiple companies to jointly analyze attack patterns across their networks without ever revealing the underlying data to one another. Similarly, homomorphic encryption allows for the detection of malicious activity by analyzing encrypted logs, eliminating the risk of exposing system-level details during monitoring.
Confidential computing also plays acritical role by ensuring that sensitive data remains protected even during processing, reducing the risk of insider threats or advanced persistent attacks. This is particularly valuable in sectors like finance, defense, or telecommunications where data breaches can have national or global implications.
By enabling secure and privacy-preserving collaboration, PETs empower cybersecurity teams to enhance detection, share insights, and coordinate defenses — without compromising the privacy or integrity of their own systems.
Compliance and reporting
Organizations use PETs to meet data privacy regulations like GDPR and CCPA while maintaining operational efficiency. These technologies enable secure data processing and analysis, allowing businesses to extract valuable insights while upholding stringent privacy standards.
A prime example is the implementation of data clean rooms — secure environments where multiple parties can collaborate on sensitive data without exposing raw information. Decentriq's data clean rooms, for instance, leverage confidential computing and operate within isolated secure enclaves. This setup ensures that data remains encrypted not only at rest and in transit but also during processing ("encryption in-use"), providing robust protection against unauthorized access. Such measures facilitate compliance with key GDPR principles, including data minimization and purpose limitation, by allowing only predefined analyses and returning aggregated results.
For a more in-depth look at how Decentriq's data clean rooms help organizations stay compliant, you can refer to our article: How Decentriq's data clean rooms simplify data protection compliance for enterprises.
How PETs enable data collaboration
In previous examples, secure data collaboration emerges as a key use of PETs, ensuring privacy while sharing insights. There are several platforms that employ privacy-enhancing technologies to enable data partnerships while maintaining the confidentiality of the data:
Data clean rooms
These are secure environments where organizations can safely collaborate on or share data while it stays protected. Depending on the data clean room provider, they will employ different combinations of PETs to build data clean rooms with privacy-preserving capabilities. Unlike clean rooms tied to specific platforms — such as those built by walled gardens or within a single tech ecosystem — independent data clean rooms are neutral, flexible, and built for true interoperability.
Here’s why they stand out:
- Platform neutrality: Third-party clean rooms are not tied to any specific media platform or ecosystem, meaning companies retain control over their data and are not limited to the constraints or incentives of a single tech vendor. This independence ensures fair collaboration and data usage terms across partners.
- PET-powered privacy: The most advanced data clean rooms leverage a combination of privacy-enhancing technologies — such as confidential computing, differential privacy, and secure multi-party computation. These tools enable precise analytics, attribution, and modeling without exposing raw data, and without compromising privacy or compliance.
- Inter-organizational trust: Collaborations involving multiple parties — such as between brands, agencies, and data providers — are far more secure when facilitated through independent data clean rooms. Each party can contribute data with full confidence that it will never be visible or misused by others, even during joint analysis.
- Regulatory resilience: Built with compliance in mind, third-party clean rooms can be tailored to meet specific regulatory needs (like GDPR or HIPAA), offering fine-grained access controls, audit logs, and data minimization by design. This is increasingly vital as data regulations tighten worldwide.
- Accelerated insights and innovation: When sensitive data can be used safely and compliantly, new use cases become possible — from privacy-safe customer journey analysis to cross-brand measurement, AI model training, and even real-time segmentation. Independent clean rooms remove the traditional trade-off between data utility and data protection.
In short, third-party data clean rooms provide the most robust and future-proof infrastructure for data collaboration. They unlock the full potential of shared data without compromising security, privacy, or compliance — and with far fewer limitations than proprietary or platform-bound solutions.
Learn how Decentriq’s data clean rooms are used by global enterprises to enable secure, privacy-first data collaboration.
Walled gardens
Closed ad platform ecosystems ("walled gardens") have their own versions of data clean rooms. A main motivator to use walled garden solutions is to do measurement without also opting-in to targeting.
However, because they control the access, rules, and data within their platform, these clean rooms pose a significant privacy challenge. Historically, they also have not integrated PETs.
Therefore, it's essential to acknowledge that there's no absolute assurance of data separation within walled garden data clean rooms. Instead, this separation relies on a specific agreement, where the technology company acknowledges that data within this environment serves a sole purpose and won't be intermingled with other data streams. The sole means of enforcing this agreement is the continuous commitment of the company, rather than any technological basis guaranteeing it.
Google Privacy Sandbox
The Google Privacy Sandbox seeks to balance user data protection with advertisers' need for insights to serve relevant ads. To achieve this balance, the Privacy Sandbox employs privacy-enhancing technologies, curbing invasive tracking like third-party cookies in favor of privacy-friendly alternatives.
The Privacy Sandbox primarily focuses on data within the web browser environment. Therefore, it may not provide the same level of data usability, collaboration, and historical data retention as data clean rooms, which are designed specifically for advanced data collaboration and analytics while preserving user privacy.
The future of privacy preservation — What should we expect?
As data privacy regulations tighten and organizations seek secure ways to collaborate, privacy-enhancing technologies(PETs) will continue to evolve. In fact, The PET market is projected to grow to $25.8 billion by 2027. Several key trends and innovations are shaping the future of PETs:
- Advancements in homomorphic encryption: Fully homomorphic encryption is progressing toward practical implementation, allowing computations on encrypted data without decryption. This will potentially enhance privacy in industries like finance and healthcare.
- Federated learning expansion: More organizations are expected to adopt federated learning, enabling AI models to train on decentralized data sources without exposing sensitive information.
- Confidential computing growth: Adoption of secure enclave technology could increase, ensuring data remains encrypted even during processing. This is particularly critical in the context of large language models (LLMs) and data collaboration, where privacy is paramount.
- Privacy-preserving AI: AI models could integrate PETs, ensuring responsible AI development without compromising user privacy.
- Regulatory alignment and standardization: Governments and institutions are working toward globally recognized PET standards to streamline compliance and interoperability.
The rapid evolution of these technologies will redefine how businesses, researchers, and policymakers collaborate securely on data. Staying ahead of these developments will be crucial for organizations seeking to maintain compliance while unlocking the full potential of their data.
Decentriq: The most privacy-preserving way to collaborate on data
Privacy-enhancing technologies are essential for organizations aiming to protect sensitive data while still leveraging its value. Decentriq stands at the forefront of this field, offering a confidential data collaboration platform that embodies the principles of PETs. By utilizing advanced encryption methods and privacy-focused techniques like confidential computing, Decentriq ensures that data remains encrypted throughout its lifecycle, allowing organizations to collaborate securely without exposing raw data.
Decentriq's data clean rooms enable secure collaboration across various industries, including media, healthcare, banking, and the public sector. These cleanrooms facilitate data analysis and model training without compromising privacy, ensuring compliance with stringent data protection regulations.
To explore how Decentriq can enhance your organization's data collaboration efforts while maintaining the highest privacy standards, visit our platform page. Ready to collaborate without compromise? Contact us to see PETs inaction.
References
Your guide to reducing wasted ad spend using first-party data
An estimated 23-56% of ad spend is currently wasted (and that’s before third-party cookies are completely deprecated). So how can brands ensure they’re reaching their ideal audiences at a time when consumers expect more personalized — yet privacy-preserving — advertising experiences than ever before?
.jpg)
Related content
Subscribe to Decentriq
Stay connected with Decentriq. Receive email notifications about industry news and product updates.